ISSN 2567-6458, 11.-12.May 2019
Email: info@uffmm.org
Author: Gerd Doeben-Henisch
Email: gerd@doeben-henisch.de
CONTEXT
This text is part of the larger text dealing with the Actor-Actor Interaction (AAI) paradigm.
HCI – HMI – AAI ==> ACI ?
Who has followed the discussion in this blog remembers several different phases in the conceptual frameworks used here.
The first paradigm called Human-Computer Interface (HCI) has been only mentioned by historical reasons. The next phase Human-Machine Interaction (HMI) was the main paradigm in the beginning of my lecturing in 2005. Later, somewhere 2011/2012, I switched to the paradigm Actor-Actor Interaction (AAI) because I tried to generalize over the different participating machines, robots, smart interfaces, humans as well as animals. This worked quite nice and some time I thought that this is now the final formula. But reality is often different compared to our thinking. Many occasions showed up where the generalization beyond the human actor seemed to hide the real processes which are going on, especially I got the impression that very important factors rooted in the special human actor became invisible although they are playing decisive role in many processes. Another punch against the AAI view came from application scenarios during the last year when I started to deal with whole cities as actors. At the end I got the feeling that the more specialized expressions like Actor-Cognition Interaction (ACI) or Augmented Collective Intelligence (ACI) can indeed help to stress certain special properties better than the more abstract AAI acronym, but using structures like ACI within general theories and within complex computing environments it became clear that the more abstract acronym AAI is in the end more versatile and simplifies the general structures. ACI became a special sub-case
HISTORY
To understand this oscillation between AAI and ACI one has to look back into the history of Human Computer/ Machine Interaction, but not only until the end of the World War II, but into the more extended evolutionary history of mankind on this planet.
It is a widespread opinion under the researchers that the development of tools to help mastering material processes was one of the outstanding events which changed the path of the evolution a lot. A next step was the development of tools to support human cognition like scripture, numbers, mathematics, books, libraries etc. In this last case of cognitive tools the material of the cognitive tools was not the primary subject the processes but the cognitive contents, structures, even processes encoded by the material structures of the tools.
Only slowly mankind understood how the cognitive abilities and capabilities are rooted in the body, in the brain, and that the brain represents a rather complex biological machinery which enables a huge amount of cognitive functions, often interacting with each other; these cognitive functions show in the light of observable behavior clear limits with regard to the amount of features which can be processed in some time interval, with regard to precision, with regard to working interconnections, and more. And therefore it has been understood that the different kinds of cognitive tools are very important to support human thinking and to enforce it in some ways.
Only in the 20th century mankind was able to built a cognitive tool called computer which could show capabilities which resembled some human cognitive capabilities and which even surpassed human capabilities in some limited areas. Since then these machines have developed a lot (not by themselves but by the thinking and the engineering of humans!) and meanwhile the number and variety of capabilities where the computer seems to resemble a human person or surpasses human capabilities have extend in a way that it has become a common slang to talk about intelligent machines or smart devices.
While the original intention for the development of computers was to improve the cognitive tools with the intend to support human beings one can get today the impression as if the computer has turned into a goal on its own: the intelligent and then — as supposed — the super-intelligent computer appears now as the primary goal and mankind appears as some old relic which has to be surpassed soon.
As will be shown later in this text this vision of the computer surpassing mankind has some assumptions which are
What seems possible and what seems to be a promising roadmap into the future is a continuous step-wise enhancement of the biological structure of mankind which absorbs the modern computing technology by new cognitive interfaces which in turn presuppose new types of physical interfaces.
To give a precise definition of these new upcoming structures and functions is not yet possible, but to identify the actual driving factors as well as the exciting combinations of factors seems possible.
COGNITION EMBEDDED IN MATTER
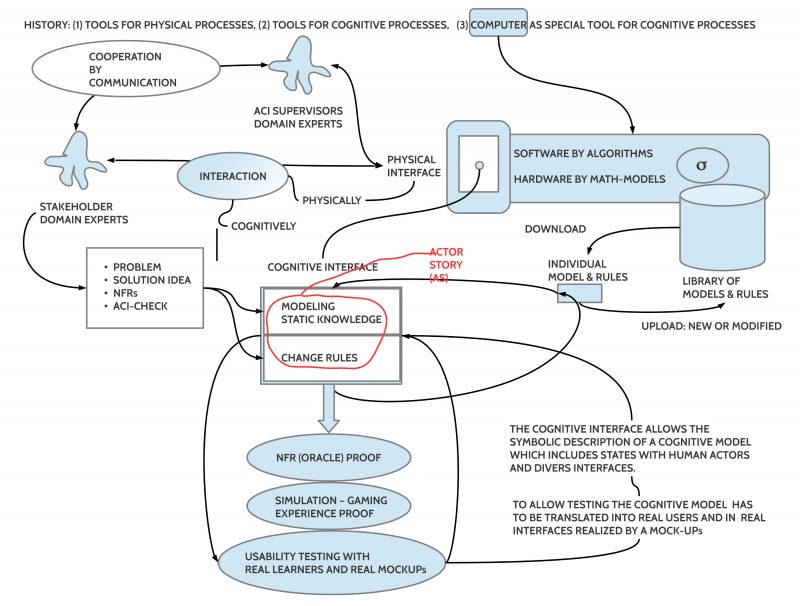
The main idea is the shift of the focus away from the physical grounding of the interaction between actors looking instead more to the cognitive contents and processes, which shall be mediated by the physical conditions. Clearly the analysis of the physical conditions as well as the optimal design of these physical conditions is still a challenge and a task, but without a clear knowledge manifested in a clear model about the intended cognitive contents and processes one has not enough knowledge for the design of the physical layout.
SOLVING A PROBLEM
Thus the starting point of an engineering process is a group of people (the stakeholders (SH)) which identify some problem (P) in their environment and which have some minimal idea of a possible solution (S) for this problem. This can be commented by some non-functional requirements (NFRs) articulating some more general properties which shall hold through the whole solution (e.g. ‘being save’, ‘being barrier-free’, ‘being real-time’ etc.). If the description of the problem with a first intended solution including the NFRs contains at least one task (T) to be solved, minimal intended users (U) (here called executive actors (eA)), minimal intended assistive actors (aA) to assist the user in doing the task, as well as a description of the environment of the task to do, then the minimal ACI-Check can be passed and the ACI analysis process can be started.
COGNITION AND AUGMENTED COLLECTIVE INTELLIGENCE
If we talk about cognition then we think usually about cognitive processes in an individual person. But in the real world there is no cognition without an ongoing exchange between different individuals by communicative acts. Furthermore it has to be taken into account that the cognition of an individual person is in itself partitioned into two unequal parts: the unconscious part which covers about 99% of all the processes in the body and in the brain and about 1% which covers the conscious part. That an individual person can think somehow something this person has to trigger his own unconsciousness by stimuli to respond with some messages from his before unknown knowledge. Thus even an individual person alone has to organize a communication with his own unconsciousness to be able to have some conscious knowledge about its own unconscious knowledge. And because no individual person has at a certain point of time a clear knowledge of his unconscious knowledge the person even does not really know what to look for — if there is no event, not perception, no question and the like which triggers the person to interact with its unconscious knowledge (and experience) to get some messages from this unconscious machinery, which — as it seems — is working all the time.
On account of this logic of the individual internal communication with the individual cognition an external communication with the world and the manifested cognition of other persons appears as a possible enrichment in the interactions with the distributed knowledge in the different persons. While in the following approach it is assumed to represent the different knowledge responses in a common symbolic representation viewable (and hearable) from all participating persons it is growing up a possible picture of something which is generally more rich, having more facets than a picture generated by an individual person alone. Furthermore can such a procedure help all participants to synchronize their different knowledge fragments in a bigger picture and use it further on as their own picture, which in turn can trigger even more aspects out of the distributed unconscious knowledge.
If one organizes this collective triggering of distributed unconscious knowledge within a communication process not only by static symbolic models but beyond this with dynamic rules for changes, which can be interactively simulated or even played with defined states of interest then the effect of expanding the explicit and shared knowledge will be boosted even more.
From this background it makes some sense to turn the wording Actor-Cognition Interaction into the wording Augmented Collective Intelligence where Intelligence is the component of dynamic cognition in a system — here a human person –, Collective means that different individual person are sharing their unconscious knowledge by communicative interactions, and Augmented can be interpreted that one enhances, extends this sharing of knowledge by using new tools of modeling, simulation and gaming, which expands and intensifies the individual learning as well as the commonly shared opinions. For nearly all problems today this appears to be absolutely necessary.
ACI ANALYSIS PROCESS
Here it will be assumed that there exists a group of ACI experts which can supervise other actors (stakeholders, domain experts, …) in a process to analyze the problem P with the explicit goal of finding a satisfying solution (S+).
For the whole ACI analysis process an appropriate ACI software should be available to support the ACI experts as well as all the other domain experts.
In this ACI analysis process one can distinguish two main phases: (1) Construct an actor story (AS) which describes all intended states and intended changes within the actor story. (2) Make several tests of the actor story to exploit their explanatory power.
ACTOR STORY (AS)
The actor story describes all possible states (S) of the tasks (T) to be realized to reach intended goal states (S+). A mapping from one state to a follow-up state will be described by a change rule (X). Thus having start state (S0) and appropriate change rules one can construct the follow-up states from the actual state (S*) with the aid of the change rules. Formally this computation of the follow-up state (S’) will be computed by a simulator function (σ), written as: σ: S* x X —> S.
SEVERAL TESTS
With the aid of an explicit actor story (AS) one can define the non-functional requirements (NFRs) in a way that it will become decidable whether a NFR is valid with regard to an actor story or not. In this case this test of being valid can be done as an automated verification process (AVP). Part of this test paradigm is the so-called oracle function (OF) where one can pose a question to the system and the system will answer the question with regard to all theoretically possible states without the necessity to run a (passive) simulation.
If the size of the group is large and it is important that all members of the group have a sufficient similar knowledge about the problem(s) in question (as it is the usual case in a city with different kinds of citizens) then is can be very helpful to enable interactive simulations or even games, which allow a more direct experience of the possible states and changes. Furthermore, because the participants can act according to their individual reflections and goals the process becomes highly uncertain and nearly unpredictable. Especially for these highly unpredictable processes can interactive simulations (and games) help to improve a common understanding of the involved factors and their effects. The difference between a normal interactive simulation and a game is given in the fact that a game has explicit win-states whereas the interactive simulations doesn’t. Explicit win-states can improve learning a lot.
The other interesting question is whether an actor story AS with a certain idea for an assistive actor (aA) is usable for the executive actors. This requires explicit measurements of the usability which in turn requires a clear norm of reference with which the behavior of an executive actor (eA) during a process can be compared. Usually is the actor Story as such the norm of reference with which the observable behavior of the executing actors will be compared. Thus for the measurement one needs real executive actors which represent the intended executive actors and one needs a physical realization of the intended assistive actors called mock-up. A mock-up is not yet the final implementation of the intended assistive actor but a physical entity which can show all important physical properties of the intended assistive actor in a way which allows a real test run. While in the past it has been assumed to be sufficient to test a test person only once it is here assumed that a test person has to be tested at least three times. This follows from the assumption that every executive (biological) actor is inherently a learning system. This implies that the test person will behave differently in different tests. The degree of changes can be a hint of the easiness and the learnability of the assistive actor.
COLLECTIVE MEMORY
If an appropriate ACI software is available then one can consider an actor story as a simple theory (ST) embracing a model (M) and a collection of rules (R) — ST(x) iff x = <M,R> –which can be used as a kind of a building block which in turn can be combined with other such building blocks resulting in a complex network of simple theories. If these simple theories are stored in a public available data base (like a library of theories) then one can built up in time a large knowledge base on their own.